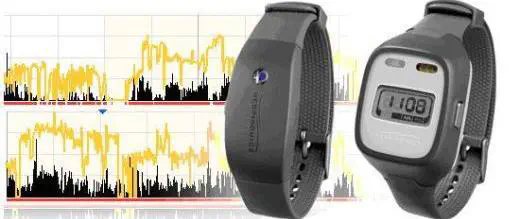
Abstract
The global incidence of depression is on the rise (Marrie et al., 2019) 1. This alarming trend highlights the urgent need for new and effective tools to detect and treat depression. Early detection and treatment of depression are crucial for improving outcomes and preventing further complications. Fortunately, recent advancements in technology have opened up new avenues for detecting and tracking depression using digital tools. One promising idea is to use motor activity data to classify the depressive state of individuals (Aminifar et al., 2021)2, (Garcia-Ceja et al., 2018)3. This approach is exciting as it has the potential to overcome the limitations of canonical depressive-symptom surveys and provide a more objective mea- sure of an individual’s depressive state.
In this study, we aim to investigate the potential of motor activity as a predictor of depressive states using motor activity (actigraph data) from (Garcia-Ceja et al., 2018)3. We will leverage several classifications models including Logistic Regression, Random Forests, K-Nearest Neighbors, Support Vector Machines, and Multi-Layered Perceptrons, and compare their performance in predicting depression based on motor activity. We will include sex and age as covariates in our analysis, as they may impact motor activity and have implications for the accuracy of our models.
We hypothesize that the random forest algorithm will outperform the linear regression model in classifying depressive state based on motor activity data (H1). We also expect that age and sex will have a significant impact on motor activity and that including them as covariates in the logistic regression model will improve its performance in depression classification (H2). Finally, we predict that the model will perform better on males compared to females due to potential differences in motor activity patterns between sexes (H3) and on individuals with severe depression compared to mild/moderate depression as their motor activity may be more significantly impacted by their depressive state (H4). Overall, this study has important implications for the development of accurate and reliable tools for detecting and tracking depression using machine learning algorithms and digital tools.
References
-
Marrie, R., Walld, R., Bolton, J., Sareen, J., Walker, J., Patten, S., Singer, A., Lix, L., Hitchon, C., El-Gabalawy, R., et al. (2019). Rising incidence of psychiatric disorders before diagnosis of immune-mediated inflammatory disease. Epidemiology and psychiatric sciences, 28(3):333–342. ↩︎
-
Aminifar, A., Rabbi, F., Pun, V. K. I., and Lamo, Y. (2021). Monitoring motor activity data for detecting patients’ depression using data augmentation and privacy-preserving distributed learning. In 2021 43rd Annual International Conference of the IEEE Engineering in Medicine & Biology Society (EMBC), pages 2163–2169. IEEE. ↩︎
-
Garcia-Ceja, E., Riegler, M., Jakobsen, P., Tørresen, J., Nordgreen, T., Oedegaard, K. J., and Fasmer, O. B. (2018). Depresjon: a motor activity database of depression episodes in unipolar and bipolar patients. In Proceedings of the 9th ACM multimedia systems conference, pages 472–477. ↩︎ ↩︎