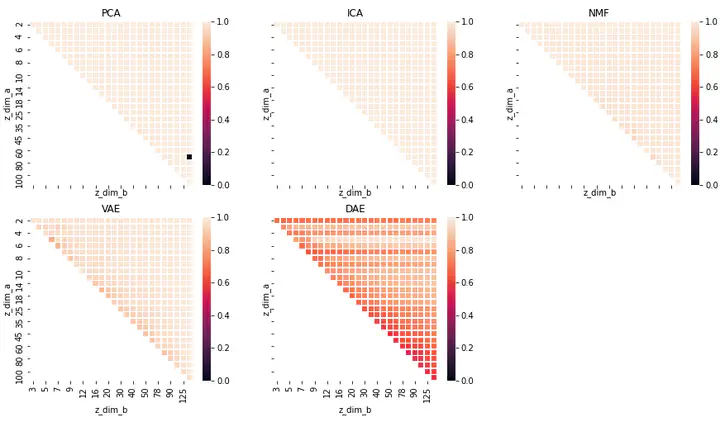
Abstract
Polycystic Ovarian Syndrome (PCOS) is a disorder caused due to endocrine dysfunction, that affects women of reproductive age. Although the aetiology of PCOS isn’t known, patients diagnosed with PCOS are generally found to exhibit elevated levels of the androgen and lower levels of progesterone.
In order to understand the pathophysiology of PCOS, we have explored PCOS gene expression data comprising 9 datasets from the NCBI GEO database. We have used unsupervised linear dimensionality reduction techniques such as Principal Component Analysis (PCA), Independent Component Analysis (ICA) & Non-negative Matrix Factorization (NMF) and non-linear dimensionality reduction techniques such as Variational Auto Encoders (VAE) & Denoising Auto Encoders (DAE) to extract biologically important signals from the data. The VAE network was trained using the binary cross-entropy loss function coupled with a Kullback-Leibler divergence penalty, while the DAE network was trained using a MSE cost function.
Our model has identified 5 genes - FAM163A, FOLR2, S100A6, AKR1A1 and MCL1, that correspond to the latent dimensions that maximally separate the PCOS data points from the control data points. These genes were found to participate in key pathways related to PCOS such as insulin secretion, vitamin and mineral absorption, insulin resistance, androgen and prostaglandins productions. Additionally, we also worked on understanding the ability of the different dimensionality reduction algorithms in identifying key features in the biological data, their stability and the similarity in the features identified by each algorithm across different latent dimensions.